Imagine a world where autonomous vehicles aren’t just concepts on paper but a seamless part of daily life. In this vision of the future, vehicles don’t just move; they think, adapt, and respond, interacting with their environment like living entities. It’s the year 2035, and the vibrant streets of San Francisco are alive with the hum of intelligent, self-driving vehicles.
Among the pioneers driving this revolution is Elena Rodriguez, a visionary AI engineer at TechNova Mobility. Her life’s mission: to redefine transportation through cutting-edge artificial intelligence. On a crisp autumn morning, Elena stands beside her masterpiece, the NovaDrive X1—an autonomous vehicle that’s not just a car but an advanced mobile intelligence. Designed with predictive analytics and sophisticated neural networks, the NovaDrive X1 represents a leap forward in human-machine interaction, redefining how people experience mobility.
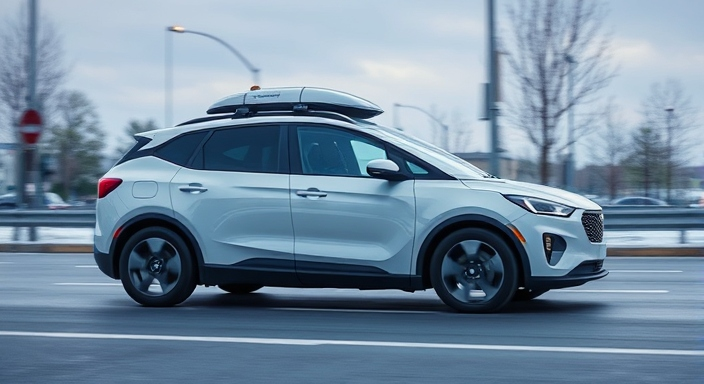
The Historical Context of Autonomous Vehicles
The idea of autonomous vehicles has fascinated humanity for decades, appearing first in science fiction and later inspiring real-world innovation. From fantastical depictions in literature to experimental prototypes, the journey of autonomous vehicles is a story of human ingenuity.
- 1920s: The earliest experiments with radio-controlled vehicles laid the foundation for autonomous driving concepts.
- 1980s: DARPA’s autonomous vehicle competitions marked significant progress in AI-driven navigation.
- 2000s: Google unveiled its self-driving car project, transforming public perception of the technology.
- 2010s: Automotive giants like Tesla, BMW, and Ford began integrating advanced driver-assistance systems (ADAS).
- 2030s: Autonomous vehicles became mainstream, with global adoption driven by advancements in artificial intelligence.
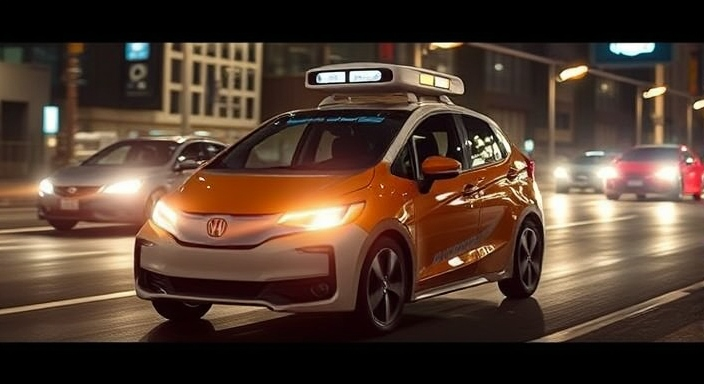
The Technological Landscape: Understanding AI’s Role in Autonomous Driving
The transformation of vehicles into autonomous entities is powered by cutting-edge technologies that enable real-time decision-making, safety, and efficiency. Here’s a deep dive into the innovations driving this change:
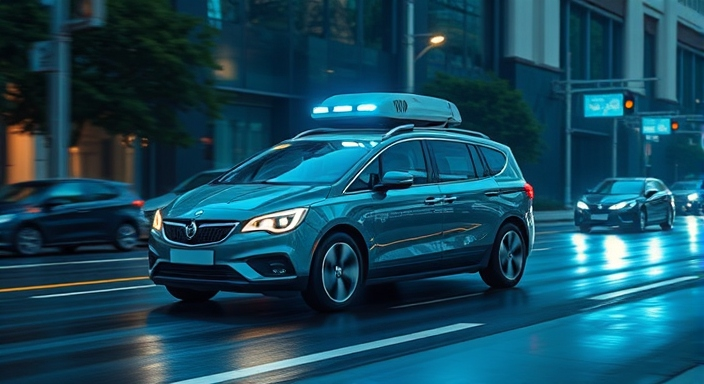
Computer Vision Technologies
At the heart of autonomous driving lies computer vision, which enables vehicles to perceive and interpret their surroundings.
- Convolutional Neural Networks (CNNs): These deep learning models allow vehicles to process and understand visual data, such as road signs, traffic lights, and lane markings.
- Object Detection Algorithms: Advanced algorithms identify and classify objects like pedestrians, cyclists, and other vehicles in real-time.
- Semantic Segmentation: This technology helps vehicles understand complex scenes by categorizing each pixel into meaningful elements like roads, vehicles, or obstacles.
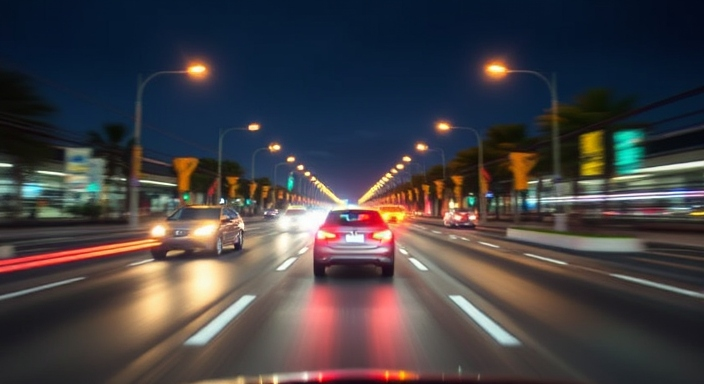
Sensor Fusion Innovations
Autonomous vehicles rely on a combination of sensors to create an accurate, multi-dimensional map of their environment. This process, known as sensor fusion, integrates data from:
- LiDAR (Light Detection and Ranging): Provides precise 3D maps by measuring distances with laser pulses.
- Radar Systems: Detects objects and measures their speed, even in poor visibility.
- Infrared Sensors: Offers reliable navigation during night-time or adverse weather conditions.
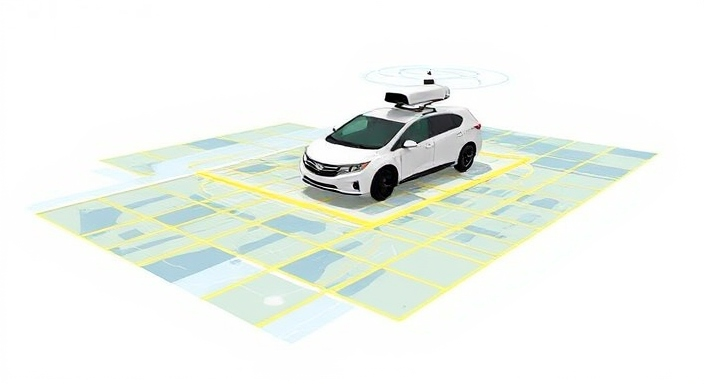
The AI Ecosystem: Tools and Frameworks
Building autonomous vehicles requires a robust ecosystem of hardware and software solutions:
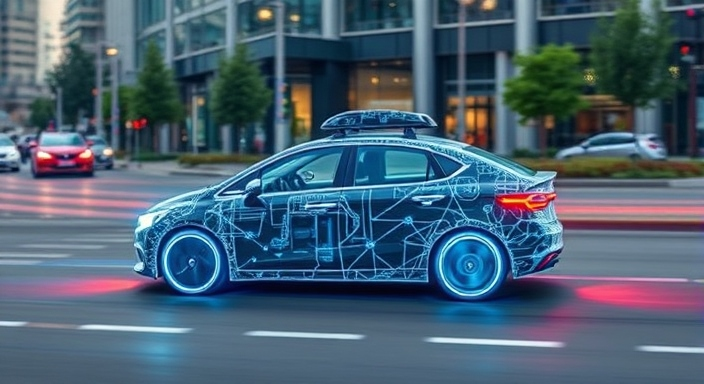
Hardware Platforms
- NVIDIA DRIVE AGX: A leading AI computing platform optimized for autonomous driving.
- Qualcomm Snapdragon Ride: A powerful processor designed for automotive AI applications.
- Intel Mobileye EyeQ: Specialized chips that process visual and sensory data efficiently.
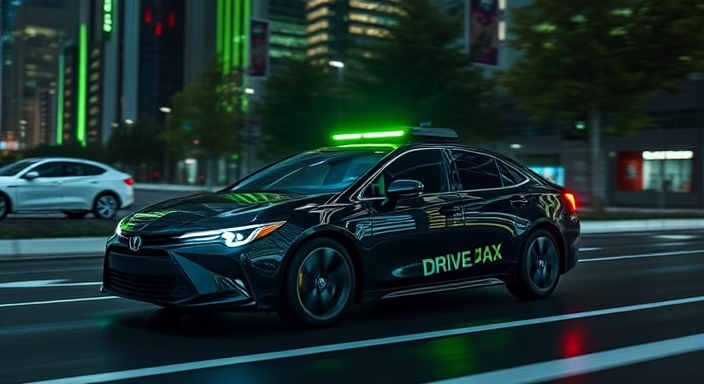
Software Frameworks
- ROS (Robot Operating System): An open-source framework for developing robotics applications.
- Apollo: Baidu’s open-source platform for autonomous driving.
- Autoware: A complete software suite for self-driving technologies.
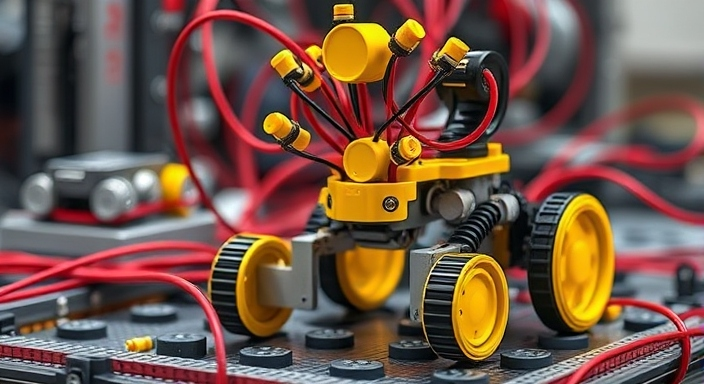
Elena’s Mission: A Technical Deep Dive
Elena Rodriguez’s creation, the NovaDrive X1, epitomizes innovation in intelligent transportation. Its architecture integrates groundbreaking AI techniques and sophisticated algorithms.
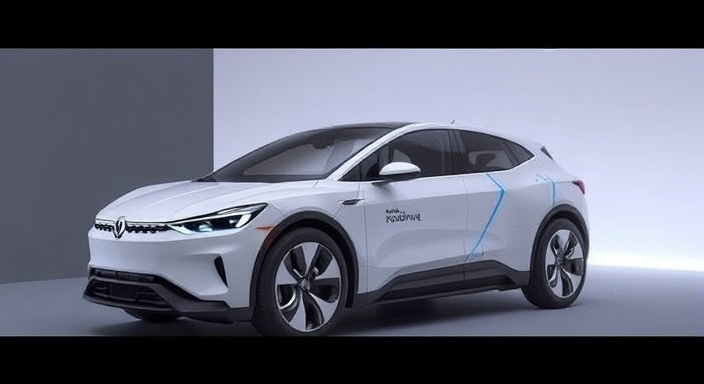
Predictive Analytics Architecture
- Machine Learning Models: Continuously trained on millions of driving scenarios to improve decision-making.
- Adaptive Algorithms: Capable of processing complex environmental data to optimize performance.
- Real-Time Risk Assessment: Enables vehicles to anticipate and mitigate potential hazards within milliseconds.
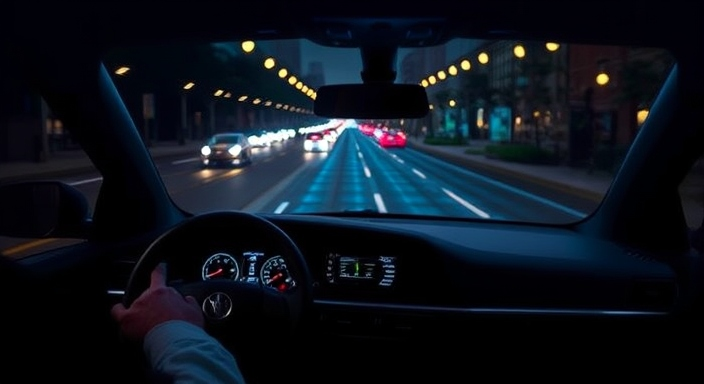
Neural Network Design
- Multi-Layered Perception Networks: Empower the vehicle to analyze vast amounts of data for accurate decision-making.
- Reinforcement Learning Modules: Improve the system’s performance by learning from its interactions with the environment.
- Transfer Learning Capabilities: Allow the vehicle to apply previously learned knowledge to new situations, enhancing adaptability.
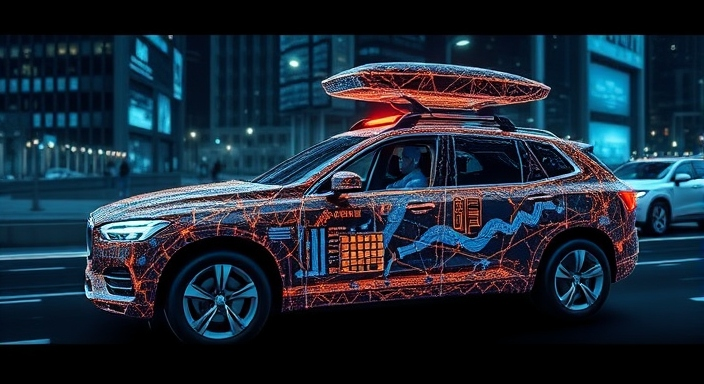
Business and Societal Implications
The widespread adoption of autonomous vehicles is reshaping industries, economies, and societal norms.
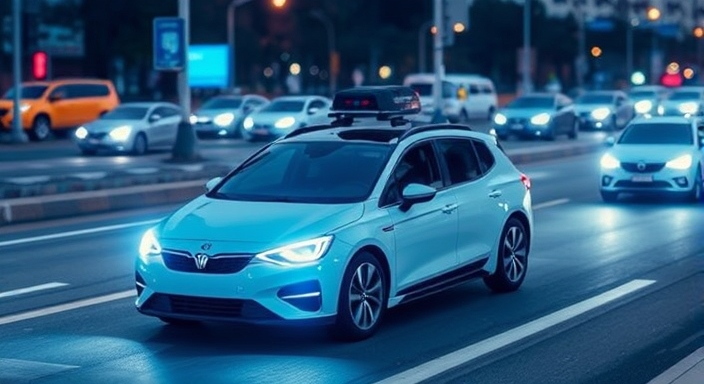
Economic Transformation
Autonomous vehicles are set to revolutionize several sectors:
- Transportation Sector:
- Logistics and delivery services will achieve greater efficiency.
- Public transportation will become more accessible and sustainable.
- Ride-sharing platforms will integrate fully autonomous fleets.
- Economic Impact:
- The autonomous vehicle market is projected to reach $2.16 trillion by 2030.
- Jobs in traditional driving roles may evolve, with new opportunities emerging in AI development and maintenance.
- Transportation costs are expected to drop significantly, benefiting consumers and businesses.
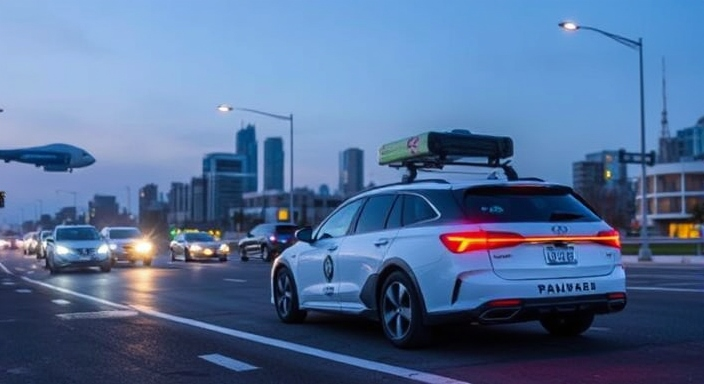
Ethical and Social Considerations
The integration of AI raises critical ethical and societal questions:
- Decision-Making Algorithms: How should vehicles handle moral dilemmas, such as choosing between passenger safety and pedestrian welfare?
- Privacy Concerns: Autonomous vehicles collect vast amounts of data. Ensuring secure and ethical use of this data is paramount.
- Accessibility: Making autonomous technology affordable and inclusive is essential for democratizing transportation.
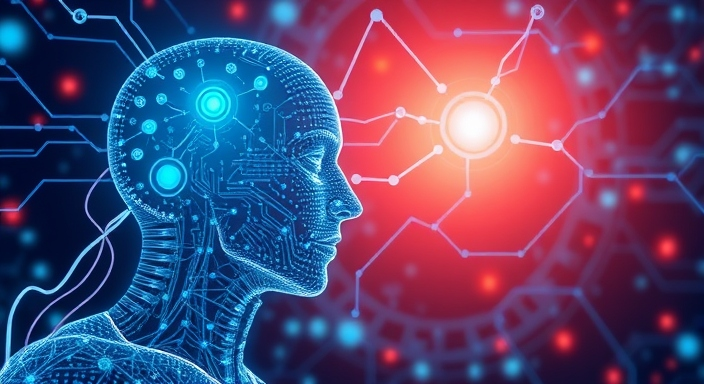
Technological Challenges and Future Innovations
Current Limitations
While autonomous vehicles have made remarkable progress, challenges persist:
- Complex Environment Navigation: Urban areas with unpredictable traffic patterns pose significant hurdles.
- Unpredictable Human Behavior: Interacting with human-driven vehicles and pedestrians remains challenging.
- Extreme Weather Conditions: Heavy rain, snow, and fog can impair sensor accuracy.
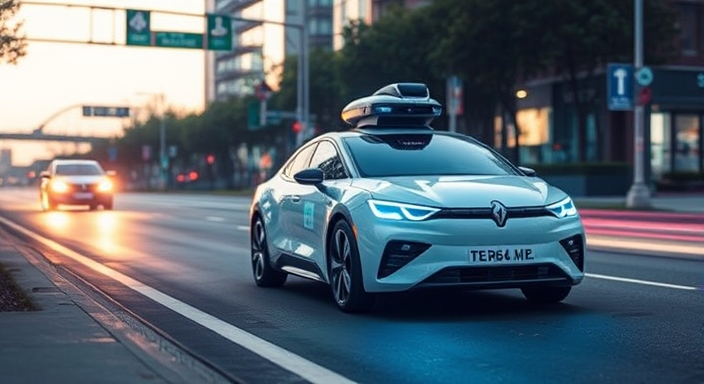
Emerging Solutions
Innovations are addressing these challenges:
- Quantum Computing: Offers unparalleled processing power to enhance decision-making.
- 5G and Edge Computing: Ensure faster communication between vehicles and infrastructure.
- Advanced Machine Learning: Improves the ability to navigate dynamic and unpredictable environments.
Global Perspectives on Autonomous Driving
The race to dominate the autonomous vehicle market has led to diverse approaches across regions:
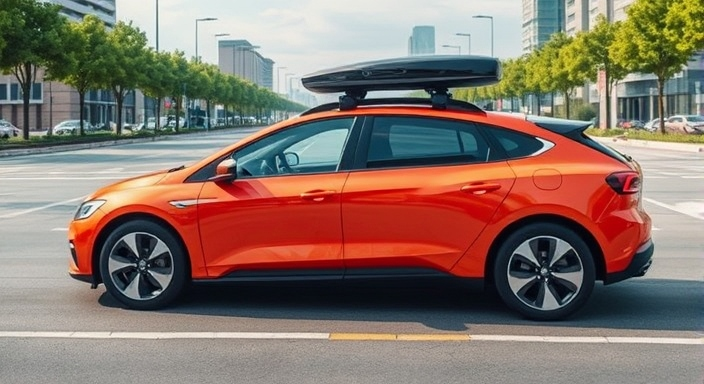
United States
- Home to Silicon Valley, a hub for innovation and AI development.
- Strong venture capital ecosystem supporting startups and established companies.
- Companies like Tesla and Waymo lead the charge in autonomous technology.
China
- Government-backed initiatives promote large-scale testing and rapid adoption.
- Companies like Baidu and Pony.ai are leveraging China’s vast population and urban landscapes for development.
European Union
- Focuses on ethical AI practices and stringent regulatory frameworks.
- Collaborative efforts across countries foster innovation while ensuring safety and compliance.
Future Outlook: Beyond 2035
As we look ahead, emerging technologies will continue to shape autonomous vehicles:
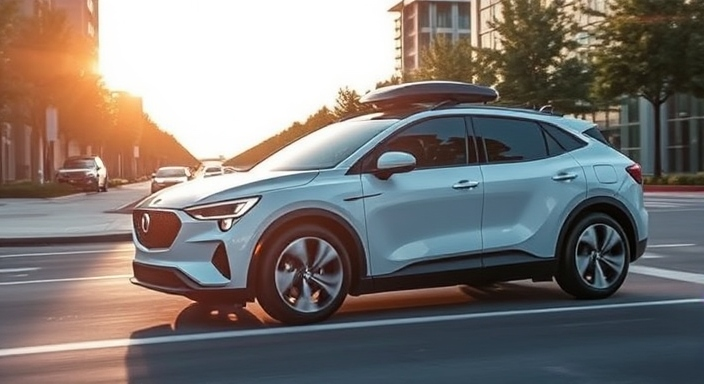
Artificial Intelligence Advancements
- Neuromorphic Computing: Mimics the human brain for unparalleled processing efficiency.
- Emotional Intelligence: Enables vehicles to respond empathetically to passengers.
- Predictive Maintenance Systems: Uses AI to anticipate and address vehicle maintenance needs.
Connectivity Innovations
- Vehicle-to-Everything (V2X) Communication: Facilitates seamless interaction between vehicles and infrastructure.
- Blockchain: Enhances data security and transparency in autonomous transportation.
- IoT Integration: Connects vehicles with smart cities to optimize traffic flow and reduce emissions.
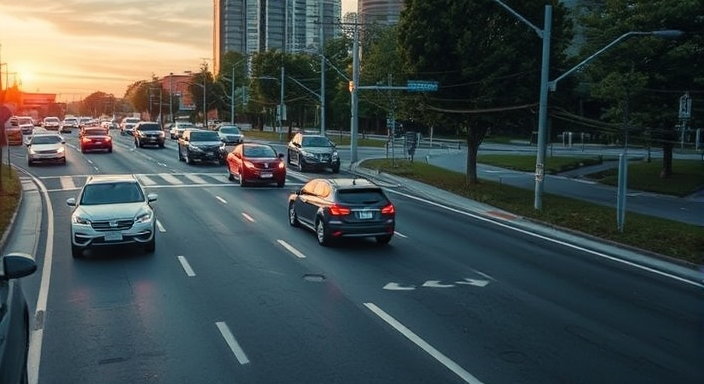
Conclusion: A Transformative Journey
Elena Rodriguez’s work on the NovaDrive X1 is a testament to humanity’s unyielding pursuit of innovation. Autonomous vehicles symbolize more than technological achievement; they represent a fundamental shift in how we perceive mobility.
The road ahead promises intelligent, sustainable, and adaptive ecosystems that enhance human potential while prioritizing safety, accessibility, and efficiency. In this transformative odyssey, autonomous vehicles are not merely tools of convenience—they are the embodiment of progress, redefining the very essence of transportation.
binance-
February 24, 2025 at 7:18 amYour point of view caught my eye and was very interesting. Thanks. I have a question for you.